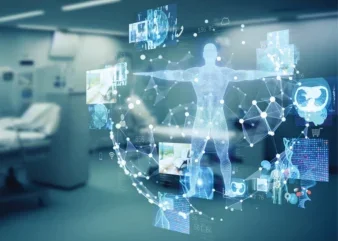
You can’t get a new medicine to market without passing it through clinical trials. But it is a famously long, slow, and terribly inefficient process. For one, there is all the documentation you need to deal with: writing the protocol and its appendages, plus reams of forms and reports. If you needed particular information from the protocol, you’d have to navigate to that document and transcribe (read: copy/paste) the bits you wanted into new downstream documents. Like pasting some text from Microsoft Word into a table in Excel. When designing the trial, you’d have to base your decisions on the data you could get – like existing literature and perhaps past trial data – making informed predictions to the best of your ability. Without a clear way to gauge the trial’s odds of success, all you can do is hypothesise. Considering how crucial clinical research is to modern medicine, you’d be forgiven for wondering: isn’t there a better way to do this?
A growing arsenal of digital tools and solutions may present an answer. By digitalising trial information, some systems can automate workflows and the exchange of protocol data. Others offer insights and analysis to inform trial design, such as assessing how likely it is to work. While these solutions are still largely in development, they have the potential to revolutionise how trials are designed and run.
Smooth data flow
“Everybody’s used to writing their experimental plan in some kind of document format,” says ex-Roche digital clinical trials consultant, Todd Georgieff. “It’s the way we’ve all been brought up.”
But the trouble with text-based documentation is that it usually needs to be transcribed into other formats to be used downstream, for instance, to feature in other files or to configure another system. Virtually every system involved in running a trial will draw from protocol information in some way – which means a lot of copy/pasting.
“The compelling vision is: what if it wasn’t a document?” says Georgieff. What if the information could be represented in a digital-first format that could be instantly accessed by other systems? This concept is known as a digital protocol.
Georgieff gives an example: when you log onto internet banking to see your balance, you are shown the numbers instantly. You don’t need to nudge someone to retrieve your statement from the bank and retype it out for you. And the numbers you see are automatically updated based on the data that is fed through the bank’s system. In a digital protocol, information could be fed to its destination automatically, allowing the instant reuse of content elements. And provided the appropriate IT architecture was in place, this functionality could be extended to version control – which may be particularly useful in managing amendments. For a large trial across multiple countries, amendments are not always approved at the same time, meaning that there might be a few different versions of the protocol floating about. “If version two is the source of truth where you are, you should really only have access to version two,” says Georgieff. A digital protocol could ensure you only see the information that applies to you. It could also enable automated workflows, such as configuring laboratory management systems based on information drawn from elsewhere. This is achieved by applying data standards – rules that determine how information should be structured so systems can talk to one another.
“You can predict the trial outcome before it starts. Then maybe you can optimise the trial in terms of adjusting the design.”
Jimeng Sun, University of Illinois Urbana-Champaign
Assessing feasibility
Digital models can provide useful insights, too. They can create databases – made up of historical trial outcomes, electronic health records, and more – and then offer analysis of that information. This can help you assess whether your trial is feasible while you’re designing it. Here, the most concrete use case we have seen to date is checking whether there are enough patients to run the trial, says computer scientist and health innovation professor at the University of Illinois Urbana-Champaign, Jimeng Sun. You would do this by plugging your eligibility criteria into a database, say of public health records and epidemiological data, to see how many patients fit the bill. You could also analyse which of your criteria are more restrictive and make any necessary adjustments. Let’s say you had a requirement that patients needed to have a value greater than 125 for a particular lab test, Georgieff explains. Yet your digital model tells you most patients who meet your other criteria actually have values of 100–125. So, you might decide to lower the required lab value to 100 to significantly increase your eligible population. “Better to know that early,” he explains.
Another approach could be to build up a library of details about your trial within a digital protocol, that you could then put together into a schedule to paint a picture of how complicated your design is. For instance, you could see the time and resources it would take to run all your patient assessments.
“If I can see that in real time, I can make adjustments as soon as possible,” says Georgieff. “This was something that users I’ve worked with have found very compelling, because they’d rather know now before they’ve made up their mind and get too wedded to a particular study design.”
Generative AI can even suggest aspects of trial design, including eligibility criteria, based on what’s been done before. Sun’s lab has developed one such model called AutoTrial, which is trained on the eligibility criteria of thousands of past trials. Much like ChatGPT, you’d input the condition you’re looking at and AutoTrial would create draft criteria – saving the time needed to go through the literature manually.
“People are all of a sudden waking up and going, ‘Maybe we should pay attention to this digital protocol thing’.”
Todd Georgieff, digital clinical trials consultant
Predicting success
Then there are systems offering perhaps the most sought-out metric of all: a trial’s odds of success. HINT, another AI model by Sun’s lab, predicts the outcome of a trial before it starts. As with AutoTrial, HINT is trained on historical trial data, from sites like clinical trial.gov. Yet because the data on trial outcomes on those websites can often be vague – they will tell you if a trial is terminated or completed but not why, for instance – HINT fills in the gaps using publicly available information, such as from news articles.
HINT collects information about the drug molecule (biochemical information about many drugs can be found online) and target disease, plus past trial protocols and outcomes. Based on what has worked before, the model can predict how your trial will fare.
“You can predict the trial outcome before it starts. Then maybe you can optimise the trial in terms of adjusting the design,” says Sun. This would be particularly useful if you had a portfolio of trials you were looking to conduct, he adds, as this could help you decide which to move forward with.
Sun and his team found that the factors most predictive of success are the condition and disease area, followed by the drug molecule itself – including its mechanism of action and its chemical features. Factors such as the eligibility criteria and trial design play a smaller role.
A third AI model by Sun’s lab, SPOT, takes this approach a step further by adding weight to trials conducted more recently. The thinking is that because scientific knowledge is evolving and expanding, newer research would be more valuable and thus lead to a more accurate prediction.
Models like this might also be used to gauge the probability of regulatory success. If you had the relevant database, you could plug in your endpoints and see how similar clinical research turned out, says Georgieff. “I could connect to a database that says, ‘Well, there have been 10 other clinical trials aimed at [those endpoints] before but only three of them have become successful label claims.”
Looking ahead
Georgieff and Sun agree we won’t have to wait too long for these digital solutions to hit the market. Tools and models that could support a digital protocol are currently in the works. For instance, the Digital Data Flow initiative by non-profit TransCelerate BioPharma and the Clinical Data Interchange Standards Consortium (CDISC) is developing a universal data standard that can be used across the industry. This will allow for the easy exchange of trial information between different systems and organisations.
And since the International Council of Harmonisation of Technical Requirements for Pharmaceuticals for Human Use (ICH) has released its new M11 guideline, which contains a standard protocol template that supports digital data exchange, more people are realising how impactful digitalising trials could be. “People are all of a sudden waking up and going, ‘Maybe we should pay attention to this digital protocol thing’,” says Georgieff. He sees digital protocol systems on the market within five years.
With predictive AI models, it could be even sooner. “I think the backbone of the capability is already there,” says Sun. “You can ask ChatGPT or any cloud type of model, and it can already give you a very informative answer.”
And ideally, one day we will be able to merge both technologies, says Georgieff. That is, having a digital protocol that can integrate predictive AI models. “[What] if I had that kind of database that could predict not only regulatory success but also clinical success as well?” he asks, “That’s the ultimate prize… The more I know, the better off I am.”