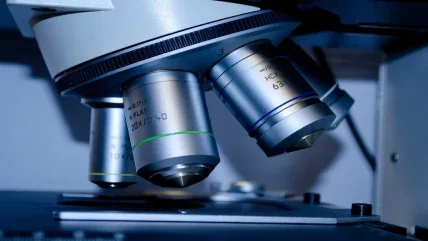
Clinical trials are designed to test the safety and efficacy of medical interventions. They were not developed to determine the economic value of those interventions, much less to optimise pharmaceutical productivity. As clinical supply chain and operations staff know better than anyone, a randomised control trial, whether it ends in success or failure, costs what it costs to get it there. Beyond that, grubby monetary considerations are supposed to be kept well away from a study’s objective pursuit of health and understanding. If only that were still an option.
‘Cost disease’ is a term well-suited to a system that spends an estimated $2.56bn to develop a new drug therapy, according to an article in the Journal of Health Economics. First used by economist William Baumol in the 1960s, the term links the increasing cost of healthcare in advanced economies to its stagnant productivity relative to manufacturing and commodity-producing industries. Productivity increases in the latter enable wage hikes that sectors such as healthcare have to match in order to retain staff and attract talent. As these sectors aren’t saving money elsewhere, however, costs climb.
Although the importance of manufacturing to the pharmaceutical industry partially shields it from this effect, one 2012 study in Nature Reviews Drug Discovery found that, despite all its technological and operational advancements, the number of new drugs approved per billion US dollars spent on R&D has halved roughly every nine years since 1950 – a drop by a factor of 80 in inflation-adjusted terms. In contrast to Moore’s Law – which describes the exponential increase in the number of transistors that can be fitted in an integrated circuit – the authors christened the trend ‘Eroom’s Law’.
Great Eroom has assigned a grim fate. In 2015, the Organisation for Economic Cooperation and Development (OECD) warned that, without reform, healthcare in advanced economies could be unaffordable by the middle of this century.
In most accounts, it is digitalisation that will prove to be pharma and healthcare’s deliverance. And yet, if that were going to be the case, one would expect the very rise of computers – and Moore’s Law with them – would be visible in the pharmaceutical industry’s productivity statistics. It’s not.
“I think it’s something of the past, this traditional trial design with huge patient populations. The uncertainties are much higher now.”
Jörg Mahlich
32–42%
The reduction in patient sampling that would have found the same result for the ProFHER trial.
ENACT
Instead, what the graphs make painfully clear is the ever-increasing failure rate of clinical research, especially in phase 3. As Jörg Mahlich, market access and government affairs lead at Miltenyi Biomedicine, points out, the vast majority of these unsuccessful trials, and particularly those run by the largest companies, look much as they might have in 1950 – too prescribed and inflexible to properly benefit from all the innovations of the intervening years. That’s despite the fact advances in precision medicine are shrinking available patient populations and increasing the difficulty of measuring clinical effects. “I think it’s something of the past, this traditional trial design with huge patient populations,” says Mahlich. “The uncertainties are much higher now.” For him, as well as a growing number of people across the industry and its regulators, it’s more considered adaptive trial protocols, rather than blind faith in technology, that will enable the industry to deal with its mounting uncertainties and address the spiralling costs of drug development.
The selling point
The need to quickly evaluate treatments and vaccines for Covid-19 has led to a notable uptick in the use of adaptive designs over the past two years, but many companies are yet to be convinced that they have benefits besides speed – particularly given the extra expenses and complexities more flexible parameters can create for logistics and operations teams. That said, recent research has shown that these may be less burdensome than previously feared: in October 2021, the University of Sheffield’s Costing Adaptive Trials (CAT) project reported that the median increase in costs for adaptive trials over traditional alternatives was between 2–4% for all scenarios, meaning resource requirements “should not be a barrier to adaptive designs being cost-effective to use in practice”.
That’s an important finding, but even before the pandemic, the FDA, EMA and other regulators were publishing adaptive pathway guidelines that stressed their potential for decreasing the cost of pharmaceutical R&D. Mahlich links his interest in boosting pharmaceutical productivity with adaptive trials to the efforts of former FDA commissioner Scott Gottlieb, noting that he was one of the first to push for more innovative trial designs in the belief that sustainable healthcare systems would be better served by lower R&D costs than tightly regulated medicine prices, which could dissuade innovation. “The major costs in pharmaceuticals are in R&D, obviously,” says Mahlich, “and bringing those costs down makes a big impact on productivity – and eventually on prices as well.” Or, as Gottlieb himself put it in 2019, “Using more modern approaches to clinical trials, we can lower the cost of developing new drugs.”
That’s exactly what Mahlich and his co-authors set out to prove in their 2021 Health Economics Review article ‘Can adaptive clinical trials help to solve the productivity crisis of the pharmaceutical industry?’. Working primarily from data published in the 2016 DiMasi et al paper that pegged the average drug development cost at $2.56bn (in 2013 USD), they estimated that greater use of adaptive trial designs could increase phase 3 success rates from 63% to between a “conservative” 70% and a “plausible” 80%, simply by reducing attrition rates. Whereas high attrition rates would necessarily doom a traditional clinical trial, adaptive designs enable investigators to re-estimate sample sizes and recruit more patients after interim analyses, enabling them to continue the study and show a treatment effect that may be of slightly lower statistical significance than anticipated but is still clinically meaningful.
In the ‘plausible’ (variation 1) scenario, Mahlich and his co-authors found that greater use of adaptive trials would reduce attrition rates from 38% to 20%, pushing overall clinical success rates up from 11.8% to 15.8%. Although the CAT project found that sample size re-estimation was the costliest element of adaptive trials – requiring a median of 26.5% more resources – using it to prevent the termination of a trial due to attrition issues can create far larger savings. Indeed, as successfully mitigating attrition rates means sponsors avoid wasting the money spent on a trial up to that point and don’t need to double their investment by restarting with a new protocol, even moderate changes in overall clinical success rates translate to very large savings. The 4% variation 1 increase would reduce the average cost of developing a new drug by 14.4%, from $2.56bn to $2.19bn. Even in the more cautious variation 2 model, costs would fall by 6.6% to $2.39bn.
In focusing only on attrition rates in phase 3, Mahlich et al’s projection may actually understate the advantages of adaptive trials. The FDA has argued that adaptive designs can yield better estimates of dose-response relationships – particularly in phase 2. Equally, Stephen Chick, Novartis chaired professor of healthcare management at INSEAD, notes that adaptive multi-arm trials can optimise how patients are allocated, and enable investigators to drop clearly inferior treatments and better focus their resources. “It’s possible to more efficiently learn when the goal is to identify the best alternative, as opposed to estimating the expected benefit of every alternative,” he explains. “So, if some alternatives are clearly inferior, based on preliminary data, you’re going to sample those less.”
For example, Mahlich and his co-authors point to the 2013 STAMPEDE trial, which simultaneously evaluated multiple treatments for prostate cancer compared with a control group, enabling the termination of treatment arms that did not outperform the common comparator. As they gather evidence, such trials can also focus on patient subpopulations that show a larger treatment effect than they do on the general populace.
Adaptive thinking can even save money for sponsors who continue to focus on traditional trial designs. As Chick explains, it’s possible to use Bayesian statistical methods to adaptively compute results in the background of a standard RCT. “If it looks like you’re headed towards a result that would say, ‘Let’s drop the trial due to futility, it’s not an effective trial,’ you can stop the trial early and save a lot of money,” he says.
Adapt and learn
Chick is a member of the management team for the UK-led EcoNomics of Adaptive Clinical Trials (ENACT) project, which is exploring the potential of a new type of “value-adaptive” protocol that incorporates cost-effectiveness considerations into the design and management of a trial – putting logistics at the core of healthcare: “This is applying process-thinking to the innovation pipeline,” he says. “It’s looking at the logistics of new technology flows and asking how we learn to get the best technology flows in place.”
In short, value-adaptive designs allow for changes to be made during a trial based on the assessment of its value for money and health. They can, for instance, enable investigators to determine whether the additional costs of continuing a trial are worth its potential benefits – incorporating relevant parameters such as trial expenses, monetary impacts and disease prevalence, which are difficult to build into a traditional trial. In a retrospective analysis of the ProFHER trial comparing surgical and non-surgical interventions for proximal humerus fractures, the ENACT team found that it could have sampled between 35% and 42% fewer patients and stopped early with the same finding, resulting in a 15% saving. Other retrospective studies showed more modest savings, and even some increases in trial length and sample sizes, but all ranked higher in expected value to the healthcare system.
“People are saying we have to be more efficient about trials,” says Chick, “but more efficient doesn’t just mean fewer patients for a given budget. It also means adapting the learning so that you get a better treatment for all the patients that are going to be following the trial. It’s looking at the net health benefit for patients after the technology adoption decision, [minus] the cost of the trial itself.”
“It’s possible to more efficiently learn when the goal is to identify the best alternative, as opposed to estimating the expected benefit of every alternative. So, if some alternatives are clearly inferior, based on preliminary data, you’re going to sample those less.”
Stephen Chick
$2.56bn
The cost to develop a new drug therapy.
Journal of Health Economics
Although value-adaptive frameworks are best suited to trials sponsored by healthcare systems, where incentives are very closely aligned with the concerns of the bodies making health technology adoption decisions, the ability to optimise trial design to create value – and to better tie investment to output – would also be extremely beneficial for pharmaceutical companies. As much as anything else, studies that do so may prove instrumental in helping the industry ensure it actually has a market that can afford its products in the decades to come. “It’s thinking about trials that inform health technology adoption decisions not as cost centres, but as value centres,” stresses Chick. A chance to reframe clinical logistics not as spending money – which any computer could do – but as creating tangible health benefits.