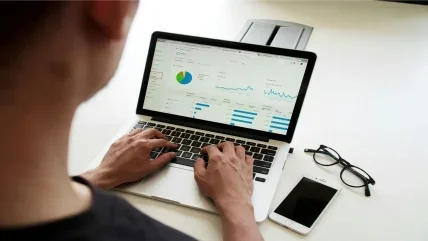
On average, it takes 14-18 months longer to run each phase of a cancer clinical trial than those in other fields. Oncology trials also tend to involve more sites, patient visits, and protocol deviations – and a lot more data collection. For instance, phase II cancer trials generate 3.1 million data points per protocol, compared to 1.9 in nononcology trials. At least, that’s what Tufts University’s Centre for the Study of Drug Development (CSDD) estimated in a 2021 report. But for those on the ground in oncology drug development, the above figures perhaps won’t come as a surprise.
Over the past decade, cancer trials have shifted in focus from traditional chemotherapy to more advanced, precision medicines, which might target a specific molecule or cancer-causing gene. This means trial designs are now more complex and that it’s tougher to find and recruit patients who are suitable for a treatment. And, when patients are enrolled, they can often be too ill to attend study visits or complete questionnaires.
All of these factors feed into a central challenge to trial success: having the data you need to determine if the treatment is any good. This is a question of not only collecting the right information, but also ensuring it’s correct and complete and putting it into an appropriate format for analysis. And with failed oncology trials estimated to cost $50-60bn each year, getting to the bottom of data management issues can be the difference between success and sizable sunk cost.
Collecting data
One of the first hurdles of good data management is ensuring you’ve collected all the information required. “It’s always difficult to get 100% of the patients to fill in the questionnaires or respond,” says Peter Hall, senior clinical lecturer in cancer informatics at the University of Edinburgh. “You can’t go back and collect that data retrospectively.”
In cancer trials, patients can be too ill to attend study appointments or even to fill out forms from home. This can create bias in the dataset, Hall explains: if a treatment made more people sick then they would be less likely to complete questionnaires, and the data collected would mostly represent those who were comparatively well.
While statistical methods can be used to try and adjust for the missing data, this doesn’t completely remove the bias, he adds. To help fill in the gaps, we might try collecting routine health information in parallel to the trial. Hall gives an example:
“There might be surrogates for poor quality of life, such as patients spending too long in hospital or taking painkillers.”
It’s currently possible to collect routine health data from the NHS for use in a trial, but it’s cumbersome. “You have to go through a whole application process to get that data, and it takes years in some cases,” says head of Data Management and Information Systems at the University of Southampton’s Clinical Trials Unit, Charlotte Stuart.
Despite calls from many in the field to make better use of real-world patient data in clinical trials, Health Data Research UK reports that just 5% of all trials in Britain used data from routine care systems between 2013-2018. In future, Hall hopes to see this become common practice.
Standardising data
In order to analyse the data you’ve collected, you need to clean it – remove and fix any errors –and put it into a standardised format. In oncology, this can be quite the task: data is often received in large volumes and from multiple sources.
Real-world data sets from the NHS tend to be in different formats due to the various ways that centres across the country record their results, while readings from CT or MRI tumour scans are down to a radiologist’s interpretation. “You get some lab results coming from genomics labs that just come via an Excel spreadsheet,” says Stuart.
Data management systems such as SAS can ease the burden of sorting through results, but often you still need to dig into them yourself, says Stuart. “It’s a very manual process a lot of the time.” Here, it helps to plan what you want your data to look like before you start collecting it, she explains. For example, you could ask the labs you’re working with to send a sample report, so you can plan how you’ll integrate all the results into one.
Sometimes, results need to be queried and followed up. In clinical trials, tumour scans must be reported on in a specific way, following a set of criteria called RECIST – which is not typically used in routine care. If you need to use an NHS radiologist who doesn’t work with RECIST – which stands for Response Evaluation Criteria in Solid Tumours – they might report in a different way, says Stuart.
When those scan results are the most important measurements to be collected in the trial (the primary outcome), teams need to go back to that radiologist and try to get them to conform to the required way of reporting. “If they don’t, then you literally just can’t use that site,” she says.
And if there’s real-world patient data in the mix, standardisation can be especially tricky, because the quality of that information can vary. To make matters worse, there isn’t currently any guidance on the best way to go about it, says Hall. “There needs to be a consensus on how to derive standardised outcomes and definitions from routine data… Routine data is inherently messy, so it would need manipulation.”
Measuring results
One of the main outcomes that oncology trials measure is the time to event – with the event usually being a progression in tumour growth or death.
5%
The percentage of all trials in Britain that used data from routine care systems between 2013- 2018.
Health Data Research UK
While measuring the latter is straightforward, there can be ambiguity when determining whether a patient is improving or becoming more unwell. Per RECIST, a therapy is considered beneficial when a tumour shrinks by 30% or more compared to its size before treatment started, while disease is said to be progressing if it grows by at least 20%.
Yet, a clinician’s assessment of a patient might not fall so neatly into those categories. If, for example, someone appeared to be getting sicker but their tumour hadn’t grown enough for them to be classed as such, a clinician could still decide that their disease had progressed, even though, according to the trial markers, it hasn’t, explains Stuart. So, which measure should be used?
For cases like these, Stuart says they have built an option into their database for “clinicianconfirmed progression”, which can override scan results. “Because at the end of the day, the clinician does probably know best,” she says. “It seems a bit harsh to say, ‘Oh, the computer says that person is not sick enough,’ when they are.”
It can also be unclear exactly when a tumour has progressed if patient scans are many months apart. “They could have progressed at four months, but you didn’t know until the six month point,” says Stuart. “When you’re trying to decide whether people in the control arm versus people on the treatment arm progressed quicker, you’ve got to have a bit of leniency in how you’re thinking about it.”
If everyone came in for their routine scans, this would all come out in the wash – but often, people miss them as they’re too ill, she adds. Here, teams might turn to real-world data to fill in the blanks.
However, routine health data regarding tumour growth probably won’t have been reported using RECIST – and at present, there’s no alternative agreed-upon measure for use in trials. “You either need to extract all the scans and get a research team to measure everything retrospectively, or you need to develop some new definition of what real world response should be,” says Hall.
More trials, more data
From 2000-2020, the number of oncology drugs in development grew by 6.5% per year – and the CSDD predicts that in the coming years, cancer trials will become even more complex and generate even greater volumes of data.
Hall hopes to see trials collect data that informs a wider variety of decision makers beyond just regulators, with the aim of more thoroughly evaluating the value of a therapy. “There’s a whole load of data missing from clinical trials…like the characteristics of the true population rather than the selected population in the trial,” he says. “And all of the health system information that’s necessary to fully assess the impact of a treatment.”
But in the meantime, we’re in good hands. For all the difficulties that come with oncology trials, Stuart notes that in her experience, they’re managed fairly well. “I think because we’ve been doing cancer trials for so long now…they’re actually run smoothly for the most part. Any issue that comes up has come up at some point before and we know how to deal with it.”