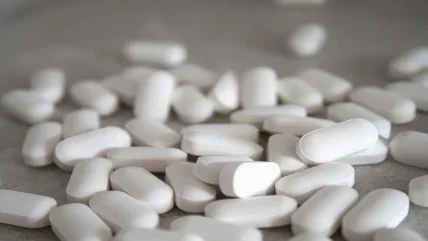
In 2018, graduate student Na le Dang made a groundbreaking discovery. A student at Washington University School of Medicine, Le Dang had been experimenting with machine-learning techniques, looking into the way certain drugs are broken down at a complex molecular level.
Her attention had been drawn to the fungal agent terbinafine, a drug that had been previously associated with several deaths from liver failure, with past researchers attributing these fatalities to a toxic compound named TBF-A in 2001. The problem was, nobody quite knew why this particular drug became poisonous once it entered the liver.
By creating an algorithm to analyse the deconstruction of these molecules, Le Dang was able to decipher the complex patterns bubbling away beneath the surface, identifying that the metabolism of terbinafine to TBF-A was an intricate process, where the compound was formed only after the first metabolic step had occurred.
While Le Dang’s discovery represented a small, but pivotal, step towards eradicating future deaths induced by terbinafine, from the perspective of the wider pharmaceutical industry, it could be a giant leap – a powerful demonstration of the potential for machine-learning techniques in spotting patterns in compounds that routinely eluded even the most experienced researchers.
Naturally, this ability to collate and analyse complex forms of information in large quantities makes AI an exciting and potentially transformative resource for life science companies, aiding data management and research departments that are frequently under-staffed and under pressure. For Joerg Stueben, a senior expert of global regulatory operations at Boehringer Ingelheim, it is these analytical capabilities that make the technology such an exciting prospect.
“AI can see things that some humans can’t,” Stueben says. “The power of this technology lies in its ability to order and interpret huge amounts of information in meaningful ways.”
Steuben has been working in regulatory operations for 24 years, starting off as deputy head of quality control for Lipoid in 1995. In his current role he is responsible for compiling and submitting detailed product records to health agencies, ensuring these reports comply with legislation in specific regions.
While historically, regulatory operators would be working with a photocopier, sending pallets of reports all over the world and waiting for lengthy periods for feedback from various health agencies, these days submissions are filed much more quickly.
For Stueben this means regularly sending detailed product information to the European Medicine Agency’s centralised database, the EudraVigilance Medicinal Product Dictionary (EVMPD) and ensuring that reports comply with the Identification of Medicinal Products (IDMP) initiative. Recently passed laws on data transparency mean that these departments are being called upon to present product details in a more standardised and structured way.
A chance for success
It’s no secret that, for many pharmaceutical companies, getting drugs to market is tough. As a recent study by Tufts Centre (CSDD) for the Study of Drug Development shows, the success rate for developing new prescription medicine stands at 12%.
To enhance their chances of success, drug companies are experimenting with AI and machine learning to try to glean insights from enormous quantities of data at their disposal.
Whereas an experienced regulatory expert might have up to 10 past submissions to draw from, public journals and cuttings, along with agency databases, yields thousands of previous reports – the online database PubMed, for example, currently has 30 million citations on biomedical literature, all of which can be drawn upon to provide a greater understanding of chemical compounds and patient reactions. At a time when staff numbers are low, but quality research is paramount, AI is being touted as a way to use company assets more efficiently.
“The time-saving capabilities are huge,” Stueben says. “A person who needs to check and read documents for about two weeks can receive the same meaningful information in about 10 minutes. Ultimately, that means our researchers are not spending valuable time reading articles that turn out to be useless.”
Natural language processing (NLP) is also providing researchers with a handy way of collecting unstructured data such as physician’s notes, pathology reports and electronic medical records, extracting insights on the properties of certain drugs and their efficacy.
This coincides with a broader move to leverage realworld data, and harness information outside clinical settings, such as health records, disease and product registries, billing data, and information recorded on health monitoring devices.
Amgen has been successful in this department, gaining approval for a form of cancer therapy based on a single-armed phase-II study, supported by evidence derived from medical records.
For Steuben, these tools offer the opportunity to extract information from health authorities, gathering regulatory intelligence on specific therapeutic areas and analysing the regulations in particular countries.
12%
Success rate for developing new prescription medicine.
Tufts Centre
“Typically, authorities will come back with followup questions, which we have a very limited time to answer,” says Steuben. “Not only are we working on responding to those questions more quickly, but trying to avoid those questions in the first place.”
With investment in machine learning increasing and breakthroughs of this magnitude gaining more traction, it is inevitable that AI is going to reshape the research and development aspect of the pharmaceutical industry. Even so, teething problems still remain.
A recent study by the CSDD and the Drug Information Association (DIA) shows that integration and technical knowledge present key challenges. Undertaken with eight pharmaceutical and biotechnology companies, the survey revealed that a lack of skilled staff, data structure and budgets constrained initiatives to successfully implement machinelearning algorithms. It concludes that regulatory constraints and data silos remain significant issues for the pharmaceutical industry that must be overcome to enable more sophisticated AI programs.
For Stueben, regulations around how to use the technology are a key area that needs to be addressed, particularly as the technology becomes more prevalent.
“On the whole, the industry needs to work more closely with regulators to come up with a framework of approval and guidance that is safe and rigid enough to enable these kinds of technologies,” he says.
In April, the US Food and Drug Administration (FDA) released a white paper proposing a regulatory framework to decide how medical products that use AI should seek approval before they go on the market, welcoming feedback on the document. So far the agency has only given the green light to devices where algorithms are frozen – meaning that they do not change each time an algorithm is used – but instead are changed by a manufacturer.
However, such regulations are not suited to algorithms with selflearning capabilities that continuously evolve, learning from past mistakes, to become more efficient.
Naturally, a key topic of discussion is how to deal with the so-called ‘black box’ problem in machine learning. In a field built on trust and transparency, the inability for advanced algorithms to show a clear thought process behind decisions is perhaps the most troubling aspect of AI application across the board.
“Ultimately, in the pharmaceutical sector, AI will gain further implementation not only because it outperforms humans at certain tasks, but because authorities can decipher when it works and why,” he says.
While the FDA and other government bodies continue to look into these quandaries, naturally the most influential developments will come from outside of the political sphere. In May, for instance, a team from Google Brain released a paper entitled ‘Human-Centred Tools for Coping with Imperfect Algorithms During Medical Decision-Making’. The paper examines an algorithmically powered user interface to manage the uncertainty of medical image classification to guide better decisions with uncertain predictions.
For now, the adoption of AI and the willingness to experiment with the technology by larger pharmaceutical companies, coupled with an increase in precision medicines and a move to harness realworld data, means that the technology is set to shape various areas of pharmaceutical research and development in the future.
In a field where roughly 90% of drug submissions fail to make it to market, where budgets are being slashed and clinical trials are costly, many pharmaceutical companies are hoping that the next blockbuster drug lies hidden in a data lake – a problem that human intuition alone cannot solve.