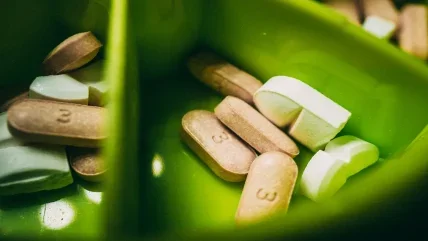
It didn’t take too long for the White House’s Office of Science and Technology to ask for help dealing with Covid-19. Given how quickly the virus spread at the start of this year, and how much damage it had already inflicted, it felt like a natural move. What is more interesting is the fact that this gesture – with its accompanying offer of a total of $10,000 in bounties – was not made to esteemed epidemiologists or vaccination experts, but to the worldwide web.
Released in March on Google’s online data science hub Kaggle, the challenge called on AI technicians with expertise in natural language processing to help solve ten questions around areas such as risk factors, genetic pre-disposition and therapeutics, which were stumping medical experts.
To help these tech wizards crack the code, more than 44,000 scholarly articles about Covid-19 and related coronaviruses were instantly made available to participants, taken from WHO. “We are issuing a call to action to the world’s AI experts to develop text and data mining tools that can help the medical community develop answers to high-priority scientific questions,” Kaggle stated.
The winners of the ten $1,000 prizes are yet to be announced, but, at the time of writing, the competition page has been viewed 2.235 million times, and 96,455 copies of the assignment brief have been downloaded. Such high numbers shouldn’t be a surprise. After close to a decade of pharmaceutical investment, and a publicity drive hailing the myriad benefits of AI – particularly with drug discovery in mind – the venture feels less like a watershed moment for the industry than an indication of where things stand.
AI has already played a well-documented role in early attempts to decipher and combat the pandemic through drug repurposing, speeding up traditional trialand- error experiments to predict how current drugs – think hydroxychloroquine and remdesivir – might treat the virus. For all the talk about new technologies, however, Professor Gisbert Schneider, chairman of the Computer-Assisted Drug Design department at Zurich’s Federal Institute of Technology, is both startled and concerned by the lack of an effective treatment against Covid-19 infections. “Where are all medicines?” he ponders. “We read tons of papers stating that AI accelerates drug discovery, but at this moment in time we have failed, as a community, to deliver an effective, efficient treatment for Covid-19.”
Schneider is quick to point out that he is not talking about vaccines here, but rather medicines and powders that could be used to subdue symptoms of the virus. In an age of fast processing and plentiful data, the lack of a breakthrough is, he maintains, a surprise. And it’s a problem for which he is more than happy to take some of the blame.
First to the party
As a leading expert in computer-assisted drug design, Schneider focuses on the development and application of adaptive intelligent systems for molecular de novo design and drug discovery. In the 1990s, he was one of the first in the field to experiment with machine learning, developing neural networks to help biochemists make insightful discoveries and design new drug molecules. At the time, it was hard to get anybody in the industry to take notice. These new technologies were at odds with how things were traditionally done.
“The pharma industry back then was extremely reluctant to look at these systems because this was not the way drugs were discovered,” Schneider says. “There was a clear dogma in big pharma. In the early 2000s, the situation was even worse.”
As Schneider admits, the bold claims made by many AI technologists – himself included – left neural networks with a lot to live up to. In the end these promising but underdeveloped systems failed to fulfil their own hype. But, 15 years on, interest is finally booming as data processing capabilities and more advanced forms of machine learning have turned AI into a powerful, albeit largely unproven, asset in drug discovery. While, using traditional methods, it can take anywhere between three and five years to unearth and test new molecular combinations prior to human studies, a slew of AI-powered start-ups are now claiming they can uncover and optimise new drugs in months – sometimes days.
Could it be, then, that the biochemists and lab technicians of the future are not humans, but complex algorithms capable of sifting through reams of data and making meaningful discoveries?
“There is a huge success rate,” Schneider admits. “In my opinion, the age-old question of whether a machine-learning or AI tool can be as creative as a chemist can be answered with a cautious yes.”
These tools, he says, can “easily compete” with teams of human researchers and chemists. Most of the time, the discoveries are indistinguishable from molecules a human mind would create. Frequently, the connections made between the roughly 1060 drug-like molecules in existence are novel and brilliant. Despite these revelations, Schneider insists that AI is not a silver bullet. Invariably, these machines are only as good as the data fed to them. Given that this information is generated by human minds and systems with an imperfect understanding of disease mechanisms, it is only natural that some AI systems struggle to process this data.
48%
The estimated percentage of scientifi c research labs across the globe that have been forced to close due to Covid-19.
The Science Advisory Board
“We read tons of papers stating that AI accelerates drug discovery, but at this moment in time we have failed, as a community, to deliver an effective, effi cient treatment for Covid-19.”
Professor Gisbert Schneider
Trust is another issue, particularly when it comes to the so-called ‘black box’ problem with AI processing. Often, advanced forms of machine learning become so inordinately complex, with their millions of layers of overlapping calculations, that a human mind cannot clearly comprehend why a decision has been made.
The man-machine
To really take advantage of these tools, Schneider advises that researchers and lab technicians adopt a collaborative approach. Rather than mysterious and untrustworthy entities, AI algorithms must be treated as partners in a discovery team.
“The team of chemists and biologists should make suggestions and ask the AI, ‘What do you think?’ In most cases, the AI will make a suggestion that the team of researchers has not thought about,” he says.
As things stand, machine-learning capabilities should help biochemists predict the effects of substances at an earlier stage of development, and suggest new chemical structures with the desired properties. This would mean that fewer ineffective substances would need to be tested. As AI continues to be harnessed to answer questions about Covid-19 and other enigmatic diseases, it goes without saying that these efforts will have a formative effect on the pharmaceutical industry, although what shape or direction these long-term changes might take is still unclear.
“I think we’re seeing changes all across the drug discovery pipeline, from idea to clinical approval. The reason for that is, over the past 20 to 30 years, R&D productivity has been declining year after year.”
Martin-Immanuel Bittner
11%
The proportion of landmark cancer papers that Amgen could validate in 2012.
Nature
With a recent survey by The Science Advisory Board estimating that 48% of scientific research labs across the globe have been forced to close temporarily because of the virus, one of its lasting legacies could be in accelerating a more remote approach to drug discovery, with AI technology and automation enabling labs able to automatically conduct and analyse biological experiments in remote robotic facilities. From London to Lahore, a raft of biotechs are now promising to automate traditionally laborious and time-consuming tasks in drug development.
For Martin-Immanuel Bittner, co-founder and CEO of biotech company Arctoris, the move is essential and long overdue. “I think we’re seeing changes all across the drug discovery pipeline, from idea to clinical approval,” he says, “[because] over the past 20 to 30 years, R&D productivity has been declining year after year.”
Even the briefest survey of the facts supports this point. According to the National Institutes of Health in the US, 80–90% of research projects fail before they are tested on humans. Moreover, an alarming proportion of research conducted on drug development is now irreproducible, plagued by unverifiable, inaccurate or inconclusive results. This problem – which stretches far beyond pharmaceuticals – is commonly known as the ‘replication crisis’. Bittner points to a survey conducted in 2012 by biotech consultants Glenn Begley and Lee Ellis at Amgen, which suggested that the company could only validate the findings in six out of 53 of its landmark cancer papers. The reproducibility figure amounted to around 11%.
“These were not dodgy journals with strange scientists,” Bittner says. “This was all widely recognised as respectable science. They found that only a very small percentage was actually reproducible. It begs the question of why we’re wasting so much time doing this menial work when the output quality is so poor.”
This led Bittner to co-found Arctoris, a company that bills itself as the first biotech to fully conduct drug trials and experiments remotely, utilising advanced forms of AI and automated robots.
It’s a big sell. Rather than restricting drug research – and, by definition, potentially exciting new discoveries – to ten or so wealthy pharmaceutical companies, Arctoris thinks it can level the playing field a little by enabling researchers to conduct these experiments remotely.
The stress is on big pharma
Quite where things go from here, however, is still uncertain. As Schneider says, while using AI and automated forms of remote working can be an immensely effective way of conceiving and experimenting with new drugs, the cost of development means that wealthier pharmaceutical companies will still control most of the market.
“We need big pharma because of all the development phases: from the first idea for a new medicine to bringing it through preclinical trials and then clinical trials, and, finally, on to the market,” he says. “That is not something a small company could do.”
Ultimately, it seems inarguable that the Covid-19 pandemic will alter the pharmaceutical landscape, but quite how drastically is anyone’s guess. What is certain is that the pandemic has placed pharmaceutical companies, and the drug development sphere more specifically, under intense levels of scrutiny. As efforts to create a vaccine continue and fatalities rise, the structures and protocols by which new drugs are conceived and created are being picked apart at a granular level like never before.
“We’re seeing changes across the whole spectrum – from idea to approval – because the industry wants to do better,” Bittner concludes. “Especially on Covid-19, [because] I think the virus has shown people how valuable it is to respond to the pandemic with new drugs and treatments, but also how fragile the whole system is.”