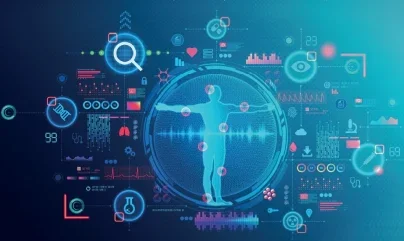
Over the past few decades, the pharmaceutical industry has experienced a concerning trend: the approval rate for new drugs has halved approximately every nine years. This phenomenon, known as Eroom’s law (the reverse of Moore’s law, where the number of components on a circuit doubles every two years) highlights the growing difficulties in bringing new medications to market. Unlike the technological advances predicted by Moore’s Law, Eroom’s Law reflects a stark reality where the innovation rate in drug development is dwindling.
The process for bringing a single new drug to market now requires billions of dollars and decades of effort. A significant portion of these resources is allocated to increasingly complicated clinical trials, which are critical to ensuring the safety and efficacy of new drugs but are also a major bottleneck in drug development. Currently, roughly only one in seven drugs that enter phase I make it through to approval.
“It’s a very interesting paradigm that we have because we have so much data that we shouldn’t be seeing that inverse,” says Jonathan Crowther, PhD, PhD, head, predictive analytics, Pfizer Research & Development, OARS. As the healthcare industry pivots to a more data-driven model, generative AI emerges as a transformative tool, offering solutions for a more efficient, cost-effective and patient-focused approach. This technology enables companies to simulate and predict various aspects of clinical trials, such as identifying the appropriate patient population, managing protocol complexity or time forecasting of the clinical trial delivery process. Crowther emphasises the major role generative AI plays in managing protocols: “We know that our protocols are getting more complex over time as an industry as we get more focused on personalised medicine and oncology, so being able to map and identify efficiencies and simplification of some of the protocols to delivery to the patient is really key.” The integration of generative AI in clinical trials has the potential to streamline these processes, effectively reducing the burden of “backend” tasks to concentrate on more meaningful work.
While the pharmaceutical industry is increasingly investing in relevant real-world data with the aim of enhancing research and patient care, the potential of this data can only be realised if it is effectively connected and leveraged across the industry. This is where generative AI, highlights Crowther, can play a massive role behind the scenes. “It’s not the sexy science, it’s the real stuff that needs to happen,” he explains. “Essentially it helps us label, connect and actually leverage all of that data.” Through retrieval augmented generation (RAG), generative AI can process and connect vast amounts of information from massive silos of data that would usually remain underutilised due to the sheer volume and complexity of data. By using contextual intelligence, generative AI can link seemingly unrelated data points to provide a comprehensive understanding of trends and patterns that would go unnoticed. “Generative AI is the solution we have been looking for to break down silos across the pharmaceutical industry,” asserts Crowther.
With the global data lake expected to reach 180 zettabytes by 2025, according to Crowther, and roughly 40% of that healthcare-related, the pharmaceutical industry is sitting on a goldmine of information. This data, encompassing everything from patient records to clinical trial results, represents an enormous opportunity to advance medical research and improve patient outcomes. But to fully capitalise on this, it’s crucial to go beyond data collection and invest in tools that can make sense of it all. Generative AI is one such tool that can visualise and interpret this data to transform raw information into actionable insights. Crowther believes that the integration of generative AI will provide “new ways and new optics or angles to look at the data that we have and how that can be applied within our sphere”.
Generative AI’s potential extends far beyond merely connecting and leveraging vast datasets, however; it also plays a crucial role in transforming the pharmaceutical industry into a more data-driven and data-savvy sector. As Crowther emphasises, “It’s really about lowering the barrier to entry for data literacy for our teams to be able to leverage that to build better solutions [and] help them build better strategies.” By making data more accessible and understandable to all members of the team, generative AI empowers healthcare professionals to utilise this information effectively, leading to improved decision-making and strategic planning. Crowther also notes that, for sponsors, the proper implementation of generative AI can significantly reduce the administrative burden associated with clinical trials. This includes time-consuming tasks of surveys and extensive documentation. By streamlining these processes, clinical sites can shift their focus to more impactful activities to improve the quality of patient care and engagement, such as building rapport with patients. This improved interaction is critical in ensuring that patients are more comfortable and engaged in their treatment processes. It also allows healthcare providers to spend more time assessing the appropriateness and effectiveness of treatments, ensuring that the right drugs are used for the right patients, in the right contexts, and at the right times.
Bias and privacy concerns
While the potential of generative AI in clinical trials is vast, it also raises significant ethical and regulatory challenges that must be carefully navigated. Crowther underscores several critical ethical concerns, starting with the issue of bias. “We have all that data, but there can also be bias in that,” he points out. For example, has there been an overemphasis on certain patient demographics at the expense of others? Crowther highlights this as a key concern: “How do we identify early datasets that might have bias and how do we remove that bias from our analysis and our predictions.” This issue is pressing because the use of historical data to build clinical models can perpetuate existing biases, leading to skewed results and potentially inequitable outcomes in clinical trials. To mitigate bias, it’s crucial to employ tools and methodologies that detect and correct these issues early in the data analysis process. One such tool is model drift, which refers to the gradual change in model performance over time as new data is collected. This concept is essential for maintaining the accuracy and fairness of AI models in clinical trials. By continuously monitoring and adjusting for model drift, researchers can ensure that their models remain relevant and unbiased, reflecting the latest and most diverse data available.
The second critical issue when considering the integration of generative AI into clinical trials is patient privacy, specifically the need to ensure that data is anonymised and compliant with HIPAA regulations. As the landscape of data security evolves, the advent of quantum computing poses new challenges and risks too. Insights from McKinsey suggest that there could be up to 5,000 operational quantum computers by 2030, significantly enhancing computational power and potentially undermining current data anonymisation techniques. “That’s a very scary prospect because organisation and anonymisation as we know it works because we have limited compute capacity,” says Crowther. “The ability to work back and break down that anonymisation is very high, but we don’t know yet so we have to be careful about things like patient safety and privacy concerns with data.” Quantum computing’s potential to decrypt anonymised data means that existing privacy protections could become obsolete, posing a serious risk to patient confidentiality. As quantum technologies advance, the healthcare industry must anticipate and counteract these threats by developing more robust encryption methods and enhancing data security protocols.
The third ethical implication centres on the concept of “explainability.” Ensuring that AI models are transparent and their predictions understandable is crucial for fostering trust and accountability within the industry. Crowther underscores the importance of this aspect, stating that ensuring that people can see and understand why models are predicting certain things is important to help level people up in the industry to understand data better. This transparency is not merely a technical challenge but a fundamental requirement for ethical AI deployment. It helps demystify AI decision-making processes, making it clear why certain outcomes are predicted, which is essential for regulatory compliance and ethical accountability. One effective approach to improving explainability Crowther points out, is the use of feature importance measures. By identifying the elements in data that significantly influence the model’s predictions and by evaluating the features, it can determine which ones contribute the most to the predictive outcomes so stakeholders can gain a clearer understanding of how the AI operates and ensure that it aligns with clinical and ethical standards.
40%
The percentage of healthcare-related data, making up a predicted 180 zettabyte global data lake by 2025.
Crowther sees a valuable lesson in how the banking industry manages ethical considerations in AI. “In banking, they actually have ethical AI consultants,” he points out, suggesting that the pharmaceutical sector could greatly benefit from a similar approach. “I think in future we should be bringing in AI ethicists … [and] I think a team dedicated to that would be a brilliant addition to the industry for every company.” By establishing teams of AI ethicists, the pharmaceutical industry can ensure that AI technologies are developed and deployed responsibly, with a keen eye on mitigating biases, protecting patient privacy, and maintaining transparency.
AI versus AI
Despite the significant potential of generative AI, its integration in clinical trials is fraught with several challenges. One major hurdle is regulatory compliance; while it is feasible for companies to leverage AI for creating documentation for regulatory agencies like the FDA and EMA, the role of the regulators themselves becomes complex. “We have come to a point where we have AI versus AI,” Crowther says. This scenario highlights a critical consideration: the role of humans. “The human in the loop is always going to need to be there, so this isn’t the case of AI taking people’s jobs – it’s about making room for meaningful work.” The challenge lies in discerning and navigating among various AI solutions, ensuring that only those which add genuine value and do not introduce unnecessary complexity are implemented. Crowther also points out the need to carefully manage interactions between different AI systems to prevent potential conflicts and inefficiencies.
Another challenge is the growing popularity of synthetic data as a viable solution to enhance the efficacy of statistical models in clinical trials. In this, Crowther underscores the importance of ensuring robust predictions by simulating data that closely resembles real-world conditions, particularly where traditional datasets are insufficient. “The challenge with that is if AI is doing it and training it, how are we 100% sure that it’s reflecting real-world conditions,” he explains. It becomes crucial to distinguish between genuinely valuable applications and mere hype to avoid pursuing uses that do not authentically improve clinical trial outcomes. This scrutiny ensures that synthetic data solutions contribute meaningfully to advancing medical research and enhancing the reliability of AI-driven insights in healthcare contexts.
Despite these challenges, the potential of generative AI is immense. “It’s a game changer without a doubt, but it is a game changer in the background,” says Crowther. He envisions a future of “just-in-time insights”, where the right information is delivered to the right people at the right time. This capability will enhance decision-making, improve patient outcomes, and streamline the clinical trial process. Ultimately, integrating generative AI into clinical trials aims to optimise interactions between sponsors, doctors, sites, and patients. Crowther believes that improving these connections will lead to superior trial outcomes, greater patient satisfaction, expedited drug development, and healthcare providers who recognise the transformative impact being made.